The Transformative Power of Machine Learning Data Annotation
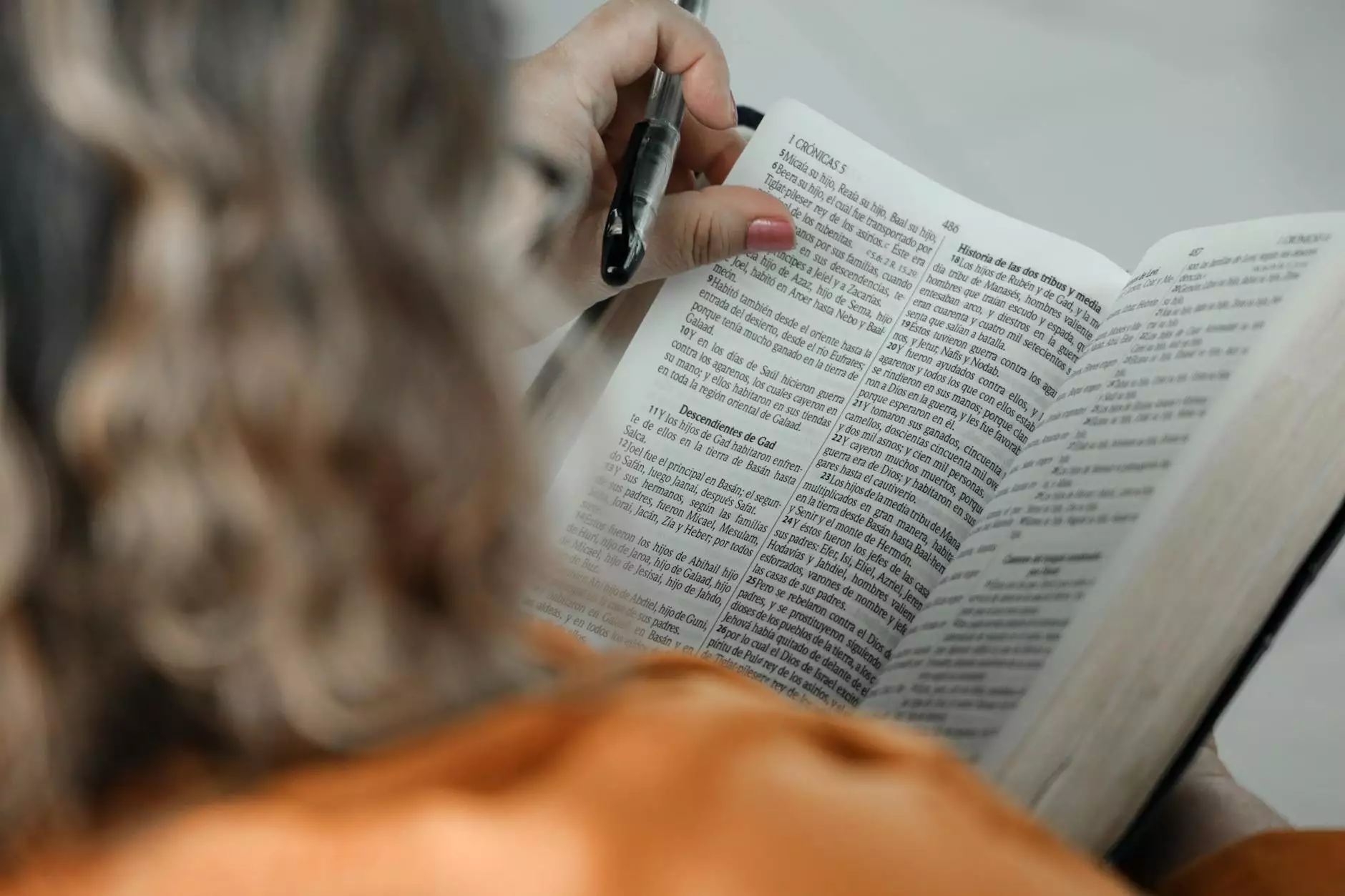
In today's fast-paced digital landscape, machine learning data annotation plays a pivotal role in advancing technologies that drive business innovation. Companies across various sectors are leveraging the power of machine learning and AI to enhance their operations, optimize decision-making processes, and create competitive advantages. This article delves into the nuances of machine learning data annotation, its significance in modern businesses, and how it can be effectively implemented to achieve substantial outcomes.
What is Machine Learning Data Annotation?
At its core, machine learning data annotation is the process of labeling and tagging data so that machines can understand and learn from it. This process is essential for training machine learning models to recognize patterns and make predictions based on data inputs.
Types of Data Annotation
There are several types of data annotation that businesses may utilize, including:
- Image Annotation: Labeling images for computer vision applications, such as autonomous vehicles and facial recognition.
- Text Annotation: Tagging words, phrases, and sentences in natural language processing tasks, including sentiment analysis.
- Video Annotation: Marking objects or actions within video footage to train models for surveillance, sports analytics, and more.
- Audio Annotation: Classifying audio clips for speech recognition and enhancement of other auditory signals.
The Importance of Data Annotation in Machine Learning
The significance of machine learning data annotation cannot be overstated. High-quality, accurately labeled data is the backbone of all machine learning models. Here are several reasons why data annotation is crucial for businesses:
1. Enhancing Model Accuracy
Machine learning models trained on well-annotated data perform significantly better than those trained on unannotated or poorly annotated datasets. Data annotation ensures that models learn the correct associations and patterns, leading to improved accuracy in predictions and analyses.
2. Facilitating Diverse Applications
From healthcare to finance, and e-commerce to transportation, machine learning data annotation enables businesses to derive insights from various forms of data, facilitating numerous applications including predictive analytics, recommendation systems, and natural language understanding.
3. Speeding Up Development Cycles
Annotated data expedites the model training process by allowing teams to quickly incorporate new data into their workflows. This speed in development means businesses can respond faster to changing market conditions and customer needs.
How to Implement a Machine Learning Data Annotation Strategy
Creating an effective machine learning data annotation strategy involves the following steps:
1. Define Your Objectives
Identify the specific goals you hope to achieve through data annotation. This could range from improving customer service via chatbots to enhancing product recommendations.
2. Choose the Right Annotation Tools
Invest in annotation tools that best suit your data type and project requirements. Options include:
- Open-source solutions: Free tools that may require more manual effort.
- Commercial software: Paid tools that typically offer more features and support.
- Custom-built tools: Tailored solutions developed specifically for your business needs.
3. Select a Method of Annotation
Decide whether to use in-house resources or outsource annotation tasks. Each option comes with its benefits and challenges:
- In-house Annotation: Better control over quality but may require additional time and resources.
- Outsourcing: Can speed up the process but may risk quality and confidentiality.
4. Ensure Quality Control
Implement robust quality control measures to ensure that the annotations are accurate and consistent. This may include reviewing a sample of annotated data or employing a multi-layer review process.
5. Iteratively Improve the Process
Continuously monitor the annotation process and the performance of machine learning models. Use feedback to refine and improve data annotation workflows over time.
Challenges in Machine Learning Data Annotation
While machine learning data annotation offers many advantages, it also comes with its share of challenges:
1. Handling Large Volumes of Data
The sheer volume of data available today can be overwhelming. Effective data management practices are crucial to handle and annotate this data efficiently.
2. Achieving Consistency Across Annotations
When multiple annotators are involved, maintaining consistency can be difficult. Establishing clear guidelines and standards is essential to minimize discrepancies.
3. Ensuring Data Privacy
Data privacy regulations, like GDPR, necessitate that businesses take careful steps to safeguard sensitive information during the annotation process. Transparency and compliance are critical.
Future Trends in Machine Learning Data Annotation
The future of machine learning data annotation is evolving with technology advancements. Here are some trends to watch out for:
1. Automation and AI-Assisted Annotation
Automating the annotation process using AI tools can significantly reduce manual labor and accelerate project timelines, making the process more efficient.
2. Crowdsourcing Annotators
Leveraging crowdsourcing platforms can help businesses tap into a larger talent pool for data annotation tasks, speeding up the process and reducing costs.
3. Enhanced Collaboration Tools
As remote work becomes increasingly common, collaboration tools that facilitate seamless communication and data sharing among teams will become vital to the success of annotation efforts.
Conclusion: The Game-Changing Impact of Machine Learning Data Annotation
In conclusion, machine learning data annotation is a fundamental component of harnessing the full potential of machine learning technologies. By effectively annotating data, businesses can significantly enhance the performance, scalability, and adaptability of their machine learning models. As technology continues to advance, those who invest in quality data annotation processes will have a distinct competitive edge in their respective markets.
For businesses in sectors such as Home Services, including Keys & Locksmiths, adopting proficient data annotation practices enables improved service delivery, operational efficiency, and heightened customer satisfaction. As we move towards an increasingly data-driven future, prioritizing machine learning data annotation is not just beneficial, but essential for sustained growth and innovation.