The Ultimate Guide to Data Labelling Tools in Business
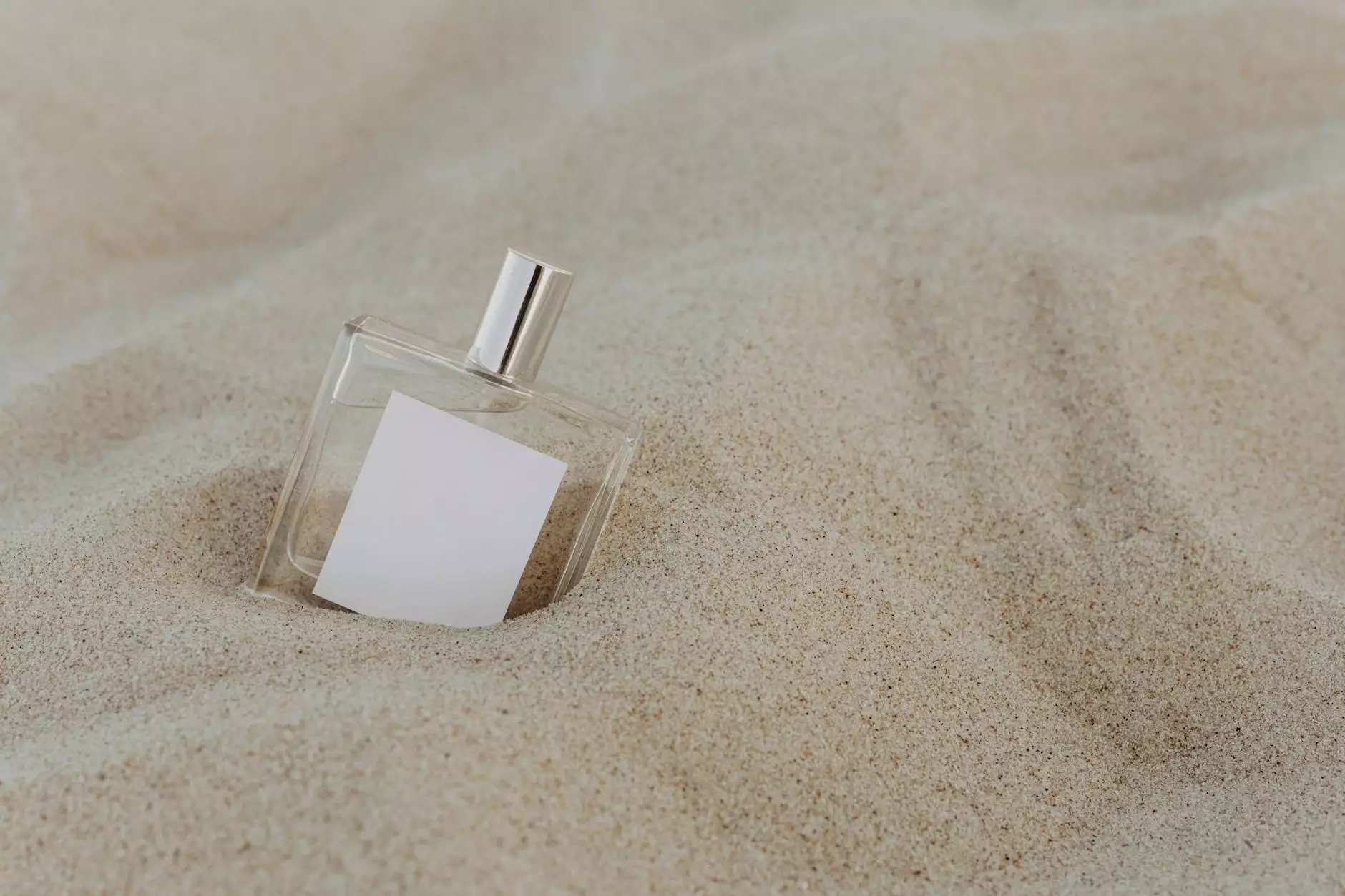
In today's fast-paced digital landscape, the ability to harness and utilize data efficiently is crucial for the success of any business. Data labelling tools have emerged as a vital component in this process, providing businesses with the means to annotate data accurately. This guide will delve into the significance of data labelling tools, their applications in various sectors, and how they can transform your business strategies.
What is a Data Labelling Tool?
A data labelling tool refers to software designed to assist users in annotating or tagging datasets for machine learning, artificial intelligence, and data analysis purposes. The process involves assigning labels to data points, enabling models to learn, recognize patterns, and make predictions based on the labelled data.
Key Features of Data Labelling Tools
- Intuitive User Interfaces: Many data labelling tools provide easy-to-navigate interfaces, allowing users, regardless of technical expertise, to label data efficiently.
- Collaboration Tools: Many platforms include features that facilitate teamwork, enabling multiple users to work on the same dataset simultaneously, thus speeding up the labelling process.
- Quality Control Mechanisms: Most tools incorporate review systems to ensure data accuracy and reliability.
- Integration Capabilities: Effective data labelling tools can integrate with other software systems, streamlining workflows and enhancing productivity.
- Multiple Data Formats Support: Supporting various data types, including images, texts, audio, and video, allows businesses to adapt their labelling efforts to different project needs.
The Importance of Data Labelling in Business
In an era where data-driven decision-making is paramount, data labelling takes center stage. Here are several reasons why it is essential for businesses:
1. Enhances Machine Learning Models
Data labelling is the backbone of machine learning. By providing high-quality, well-annotated datasets, businesses can train their models more effectively. This leads to improved accuracy and performance, which is critical for applications such as predictive analytics, natural language processing, and image recognition.
2. Informs Better Business Decisions
Quality data leads to informed decision-making. With properly labelled data, businesses can extract insights and trends that guide strategic planning and operational improvements.
3. Optimizes Customer Experience
Data labelling tools allow businesses to analyze customer interactions and feedback. By understanding customer sentiments through labelled data, companies can tailor their services and products to meet customer needs effectively.
Applications of Data Labelling Tools Across Industries
The flexibility of data labelling tools enables their use across various sectors:
1. Healthcare
In the healthcare sector, data labelling is critical for developing diagnostic tools and medical imaging analysis. For example, annotating images of medical scans helps in training AI systems to detect anomalies, leading to quicker diagnoses.
2. Automotive
The automotive industry relies on data labelling to improve autonomous driving technologies. Annotated images from sensors and cameras are vital for training self-driving cars to recognize road signs, pedestrians, and other vehicles.
3. Retail
In retail, data labelling can enhance customer experiences by powering recommendation systems. By analyzing labelled purchase data, businesses can suggest relevant products, increasing sales opportunities.
Choosing the Right Data Labelling Tool
When it comes to selecting the best data labelling tool for your business, consider the following factors:
1. Ease of Use
Choose a tool that offers an intuitive interface. This will minimize training time and help your team get started quickly.
2. Scalability
Your chosen tool should be able to grow with your company. As your data needs increase, the tool should accommodate more complex data sets and additional users.
3. Accuracy and Quality Assurance
Look for tools that have built-in quality control features. This ensures that the data you label is accurate and reliable, which is crucial for machine learning models.
4. Cost-Effectiveness
Analyze the pricing models of different tools. Some offer pay-as-you-go services while others may be subscription-based. Choose what best fits your budget while ensuring quality.
5. Customer Support
Reliable customer support is essential, especially when integrating new technology into your operations. Look for tools that offer excellent user support and resources.
Best Practices for Effective Data Labelling
By adhering to best practices, businesses can maximize the efficacy of their data labelling efforts:
1. Establish Clear Guidelines
Define clear labelling guidelines for your team. This includes specifying what labels to use and how to apply them consistently across different data points.
2. Use a Diverse Workforce
Diversity in the labelling team can help reduce biases in the data. Different perspectives can lead to more comprehensive labelling practices.
3. Regularly Review and Update Labels
As your business evolves, so may the relevance of certain labels. Regularly reassess your labelled data to ensure it remains applicable to your current needs.
Future Trends in Data Labelling Tools
The field of data labelling is constantly evolving. Here are some future trends to watch:
1. Automation and AI Integration
AI is set to revolutionize data labelling with automated tools that can label data faster and potentially with greater accuracy than humans alone. This could significantly reduce time and costs for businesses.
2. Increased Use of Crowdsourcing
Crowdsourced data labelling is gaining traction, where businesses employ large groups of individuals online to label data. This method provides scalability and cost-effectiveness.
3. Enhanced Collaboration Tools
Ongoing enhancements in collaboration features within data labelling tools will streamline how teams work together, thus increasing overall efficiency.
Conclusion
In conclusion, data labelling tools are indispensable in the modern business environment. They not only facilitate the training of AI and machine learning models but also provide businesses with insights that enhance decision-making and operational efficiency. By investing in the right data labelling tools and adopting best practices, companies can stay ahead of the competition and leverage their data more effectively. With advancements in technology, the future of data labelling promises even greater improvements and opportunities for businesses across various industries.